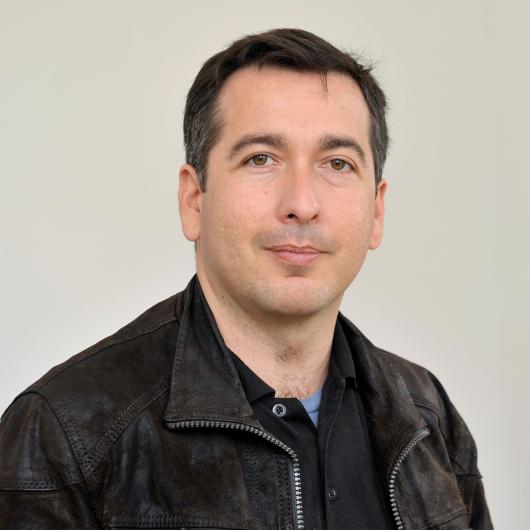
Dr Gerald A. Corzo is a senior researcher with extensive knowledge on ICT and its applications in water resources problems. He has completed civil engineering with studies in teleinformatics, and he has a Master and PhD in hydroinformatics applications using machine learning models. Gerald has postdoctoral experience using Big Data analytics for Climate Change extremes at Wageningen University.
His primary areas of expertise are in hybrid machine learning and process-based modelling. He has worked with spatiotemporal analysis of extreme events under climate change scenarios, and contributed to the area of pattern recognition and tracking of extremes.
Gerald produced more than 40 high impact journal publications, various book chapters, journal special issues and book editorials. In 2012 he won the Tison award from the International Association for Hydrological Science (IAHS). He participated in various EU and international projects and developed a trajectory in spatial statistics. He coordinated the statistics of the Climate change inventory of adaptation and mitigation actions for Latin-America, presented at the World Water Forum in 2012. He also wrote the report 43 on future extremes from the WATCH EU forcing data set and a big data analysis of the Climate model scenarios.
In 2020 he coordinated the analysis of climate change for the national atlas of climate change in the Dominican Republic. Currently Gerald is focussing on AI and spatiotemporal analysis of socio technological and water resources problems.
One of his recent projects focuses on Natural processing language for understanding and finding causation of decision making from cloud data. Part of the development in the area of data collection use of citizens science and mobile teechnologies. As part of the concept of citizens involvement an “intelligent” serious game was developed to collect data and explore knowledge and behaviour in citizens. As project leader, lecturer and research supervisor he has the opportunity to cooperate with institutes around the world, building a large network for science and development cooperation.
Publications
A complete list of publications can be found in ResearchGate.