Remote Sensing for Agricultural Water Management
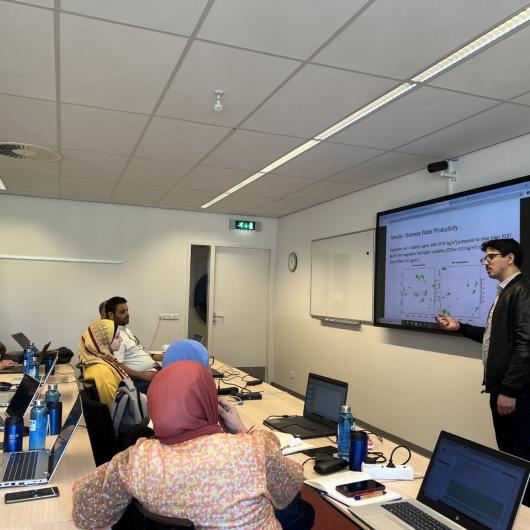
Develop skills to use remote sensing data for estimating high resolution agricultural water use (evapotranspiration) and biomass production for your own area of interest and utilise this data for water productivity and irrigation performance assessment.
For whom?
Young and mid-career professionals, engineers and technicians and academics involved in irrigation system management in various government and non-government organizations.
Prerequisites
Certificate from self-paced online course on python essentials for geospatial analyses.
AND
Proven work experience in GIS and/or Remote Sensing
Required skills: Preferably QGIS (or other GIS software); programming (preferably python), Microsoft Office (Word, Excel, PowerPoint)
Learning objectives
- Apply a remote sensing based approach to estimate high resolution actual evapotranspiration and biomass production
- Conduct irrigation performance assessment at a scheme level using indicators such as water productivity, adequacy, beneficial fraction and equity
- Explain the use of remote sensing in assessing water use and biomass production in agriculture
Course content
Monitoring and assessing land and water use in agriculture is key to understand the resource usage and plan interventions for ensuring sustainable use. Obtaining such information using field based methods is challenging due to several factors such as cost, practicality in terms of coverage, maintenance for long term support. Remote sensing offers a reliable alternative in monitoring the key variables continuously and at an unprecedented scale.
In this short course, application of remote sensing techniques to assess the performance of irrigation by estimating water use (Evapotranspiration) and agricultural production (crop yield/biomass) are covered. The course is designed to allow participants to apply the skills developed on their own case study. Participants will learn from data acquisition to retrieving key information on land and water use through lectures and connected hands-on exercises.
Topics
- Overview of remote sensing applications in agricultural water management
- Remote sensing based methods to compute evapotranspiration using satellite data
- Remote sensing based crop yield and water productivity assessment
- Remote sensing for irrigation performance assessment
Throughout the course we will be using open source softwares and libraries and open access data.
The course will be evaluated through formative and summative assessments. Formative assessments are designed through quizzes and reading/reflection exercise. Two reports containing results, analysis and reflection have to be submitted as final summative assessment to obtain the academic credits and transcript.
Additional information:
- Chukalla, A. D., Mul, M. L., van der Zaag, P., van Halsema, G., Mubaya, E., Muchanga, E., den Besten, N., and Karimi, P.: A framework for irrigation performance assessment using WaPOR data: the case of a sugarcane estate in Mozambique, Hydrol. Earth Syst. Sci., 26, 2759–2778, https://doi.org/10.5194/hess-26-2759-2022, 2022.
- IHE Delft, 2024. WaPOR Irrigation Performance Assessment (WaPORIPA) github repository.
- IHE Delft, 2024. MOOC Python for geospatial analyses using WaPOR data
- FAO, 2024. PyWaPOR repository.
PyWaPOR training in Delft on the use of remote sensing for agricultural water management
IHE Delft Institute for Water Education, a key partner in the WaPOR project, offered a short intensive course (workload of 84 hours) on Remote Sensing for Agricultural Water Management in its premises in Delft, The Netherlands, between March 3 and 14 March 2025.
Course Coordinator
Marloes Mul
Associate Professor of Water Resources Management
Related
Student testimonial ·
Faazla Iqbal: an engineer studying water to serve humanity
Faazla Iqbal from Pakistan studies water to deepen her connection with the field and contribute meaningfully to humanity. With a background in electrical engineering, Faazla came to the Netherlands to pursue a Master's in Water and Sustainable Development, specialising in the Water-Food-Energy track and the Hydroinformatics profile.
News ·
2024 reflections: Water and Development Partnership Programme
The year 2024 has been an inspiring, yet challenging year for our Water and Development Partnership Programme. As it comes to an end, we reflect on the partnerships, experiences and achievements that shaped our efforts and impact over the past months.
News ·
Rain of hope: Yemen's fight for water in a time of war
Yemen is one of the most water-scarce countries in the world, and a decade of relentless civil war has pushed it to the brink of an acute water crisis. The conflict has wrecked important water infrastructure and ruined traditional farming in cities—an important food source known as Maqashim. Families forced to flee their homes due to the war are adding to the strain and there isn’t enough clean water for both domestic and agricultural use. Each day, the need for sustainable water solutions becomes more urgent.